This is a special retrospective by AI Resident Lamtharn (Hanoi) Hankatrul, who we’ve had the pleasure of working with for the past two years.
Tone Transfer is a fun and creative tool for sound transformation released a couple of months ago by Google. One of my favorite transformations I designed for the experience is the Indian Carnatic singer transformed into a flute. Have a listen for yourself! Tone Transfer can re-render a Western concert flute to exactly follow the meend, or melodic twists and turns from Carnatic singing. Over my last two years as a Google AI Resident, I’ve learned how machine learning (ML) can open up new forms of musical and cross-cultural pollination. Transcultural technologies empower cultural pluralism at every phase of engineering and design. While this principle is far from the norm in ML, a number of projects explore how machine learning that is transcultural can transform technical, artistic and social dimensions of music and culture.
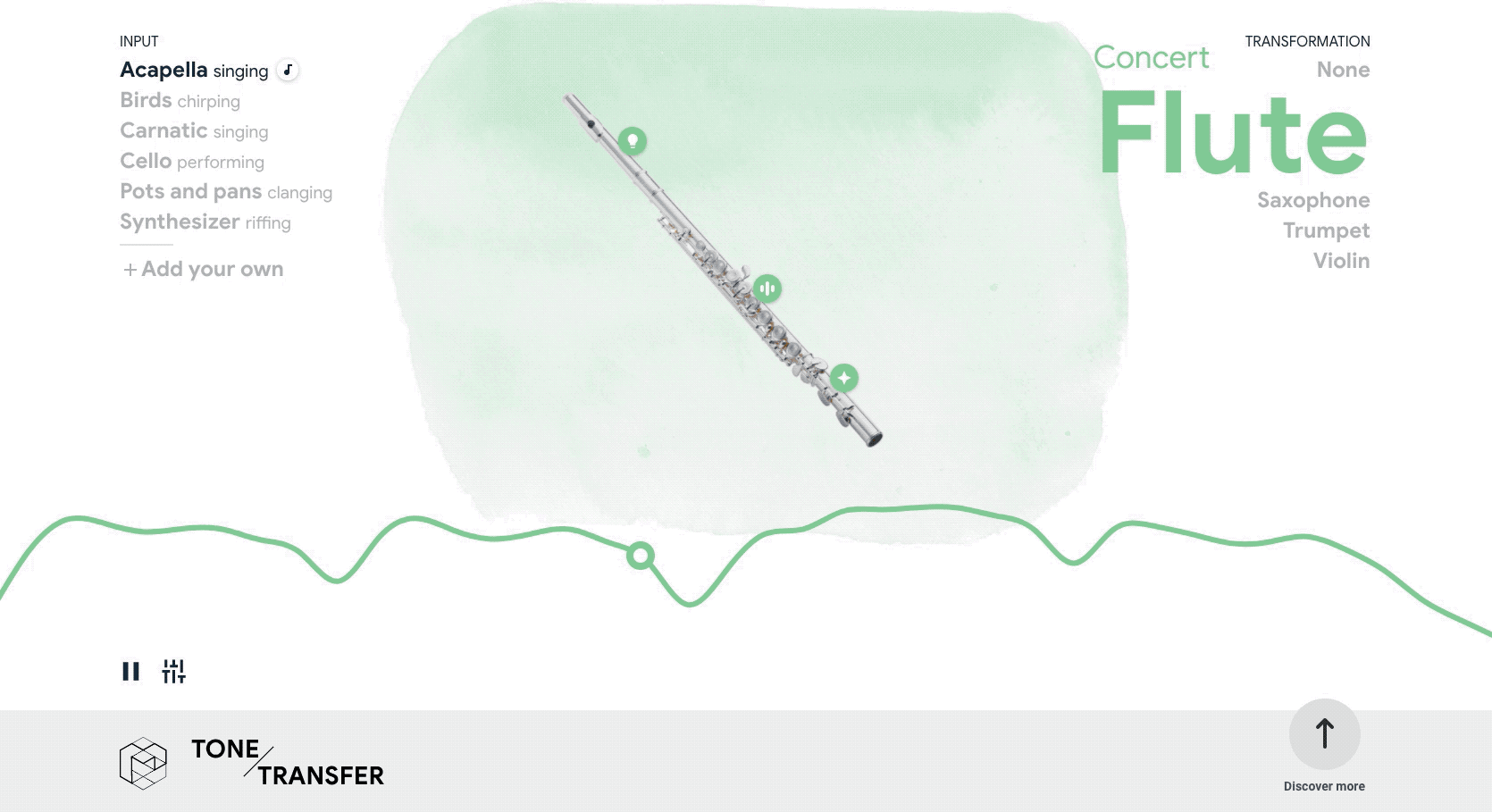
Before joining the Magenta team for my AI Residency, I had been incubating the idea of transcultural technology during a unique gap year. I was apprenticing with luthiers in my home country of Thailand, learning how to build musical fiddles like the Northern Thai Salo. I was taught how to chop coconuts and carve wooden pegs exactly like my ancestors did hundreds of years ago. My hands-on experience highlighted how new technologies are often applied within the cultural boundaries of their inventors. In instrument making, techniques like 3D printing and materials like carbon fiber have largely been limited to Western instruments such as the violin. What would my own traditions look like when re-imagined through science and design? I set out to develop Fidular, a modular and cross-cultural instrument fusing classic woodworking with modern 3D printing. Fiddlers and craftsmen could quickly build a hybrid fiddle made from resonators and strings from across the Asia-Pacific and Middle East. The finished transcultural design received international recognition, and inspired me to think about ways the power and scalability of other technologies, such as ML, could widen the scope of transcultural engineering and design.
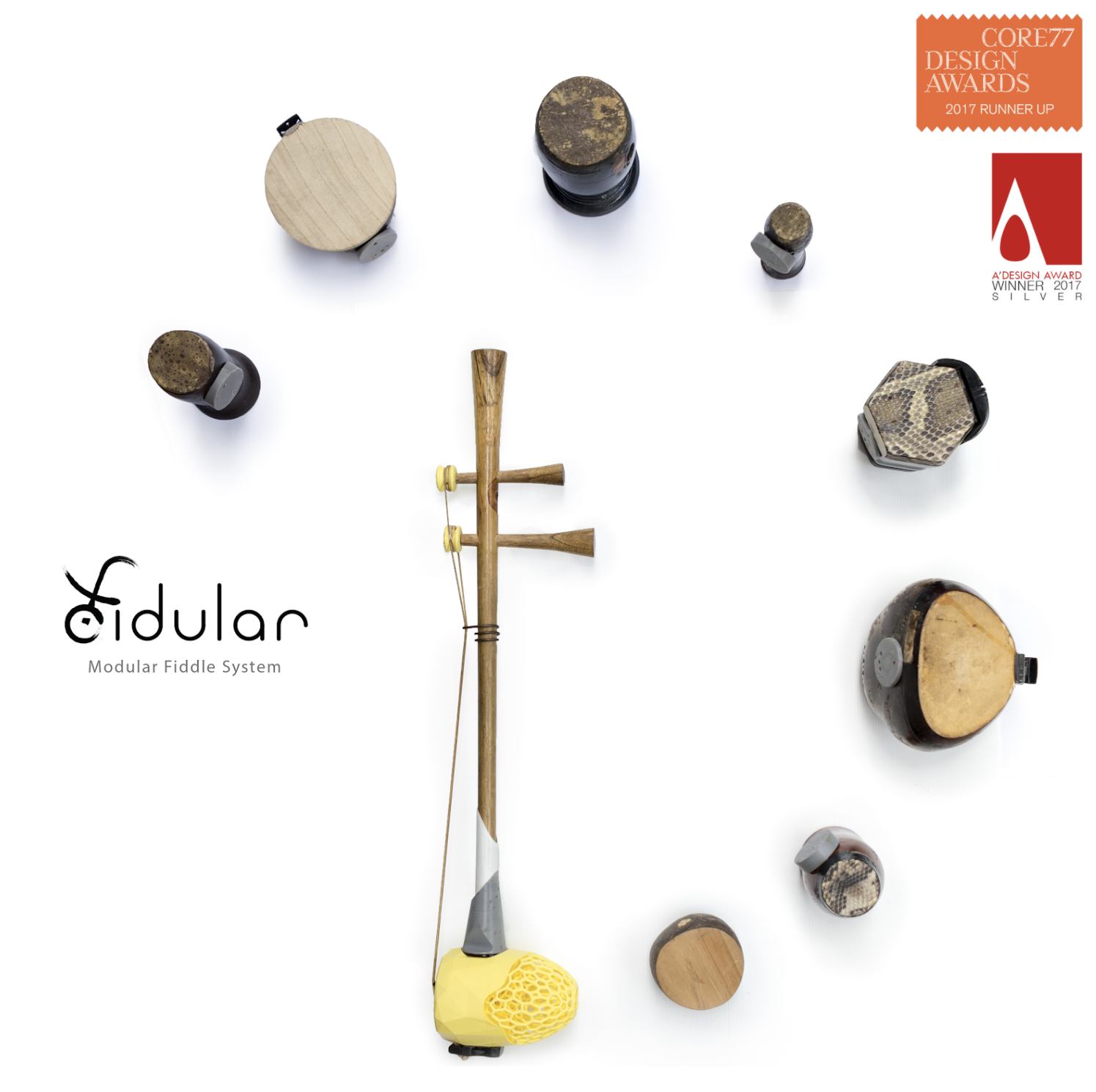
Examining bias is key to building transcultural techonologies. By definition, ML systems are biased; they “have a viewpoint” and use mathematical bias to make decisions. This bias can come from two places. Model bias refers to choices a researcher makes which encode properties about the data. Dataset bias refers to how the data was collected and its composition. As my research mentor Jesse Engel often stressed, while these choices are essential to make a model work, they bake in limitations by definition. It’s important to make these choices clear and explicit in our musical context.
Many AI music models embed structure from Western classical music (i.e. model bias). Melodies are divided into one of twelve pitches in an octave; like a piano’s black and white keys. In Thai classical music, the same octave is divided into seven pitches. Many of these models thus cannot be applied to Thai melodies. When I attempted to address this by encoding rules of Thai music, I hit a second problem. Datasets of music from Thailand and around the world that are labelled, high quality and have appropriate licenses for training are difficult to find (i.e. dataset bias). At this point, the problem becomes more than mathematical in nature, and tied instead to larger structural issues: notably the white, male, and colonial history of music and ML scholarship. Systemic themes of gender, race and cultural representation affect all areas of AI research, from natural language processing (NLP) to computer vision (CV). As a Southeast Asian who grew up outside the Western hemisphere, I believe addressing inequalities in AI requires a convergence of coordinated responses between cultures from across scientific and social spectrums.
It is from this perspective I am most proud of my AI Residency projects: DDSP, Sounds of India and Tone Transfer. Beyond empowering cross-cultural transformations, DDSP models thrive in low-data environments typical of underrepresented music. With as little as 15 minutes of data, you too can train your own model of lesser-known instruments. DDSP models are fast and lightweight, capable of rendering audio in-browser within seconds and with on-device privacy. In Sounds of India, it enabled us to celebrate Indian classical music through AI on millions of mobile phones simultaneously. We trained our deployed models directly on short, commissioned recordings from three instruments: the Bansuri, Shehnai and Sarangi. This is a giant leap from earlier work during my AI Residency, where models were limited in scope to Western datasets, requiring hundreds of hours of recording data and powerful computing resources only available at places like Google.
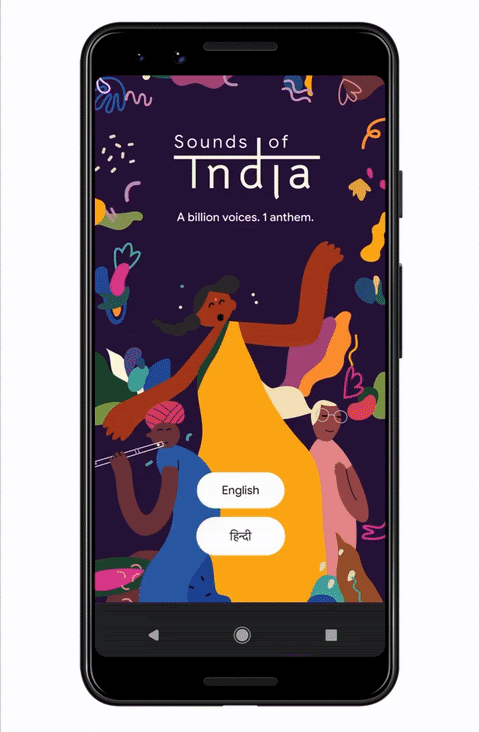
I was excited to apply this approach to as many musical cultures as possible, much like with Fidular. I recorded instruments such as the Chinese Dizi and Persian Setar from Googler volunteers and aimed to open-source them all. One day, I started playing with models trained on the Chinese Guqin zither. The transformation from voice wasn’t particularly good. When I played it to my Chinese colleague from our partner AIUX design team, his facial reaction - a priceless mix of skepticism and aversion - made me reevaluate my position entirely.
Machine learning models are never 100% accurate. Cultural ownership complicates this further; especially for underrepresented ones. We were worried non-Chinese listeners hearing the Guqin for the first time through our technology could form incorrect impressions of the instrument and, in turn, Chinese classical music. We decided not to open source these models in Tone Transfer or the DDSP colab notebook. In Sounds of India, our team launched the experience on Indian Independence Day in partnership with Prasar Bharti, India’s public sector broadcaster. This ensured our models of Indian instruments were aimed at local audiences familiar with their original sounds.
The context of a model’s creation matters. Like other artefacts of culture such as national anthems, fabrics and symbols, models are cultural distillations that should be treated with care and respect. DDSP is much like a modern re-incarnation of sampling, a musical process which gave birth to new genres like hip hop. But it could also set loose a new form of AI-powered cultural appropriation. Our teams, from Magenta to AIUX, firmly believe this level of technological-cultural sensitivity is paramount to ensuring we do not appropriate, cause cultural harm, or implant users with wrong expectations through machine learning.
So far, the responses to both Tone Transfer and Sounds of India have been terrific. Check out videos by Youtubers Andrew Huang, Adam Neely and artists like Mija and TEMS where Tone Transfer is pushed to its limits in fun and musical ways. Community generated content and comments for Tone Transfer and Sounds of India ring with excitement for the technology. It brings great joy to our teams and myself to see our careful considerations of culture, music and machine learning having positive impact. These experiences have left me feeling more energized to pursue transcultural principles in broader domains of technology and society. If these ideas resonate with you, please reach out and consider implementing modules that address these topics through the open-source Magenta Github!
Acknowledgements
A big shout out to my research mentor Jesse Engel for the multiple revisions and help refining the narrative of this piece. Many thanks to Googlers who mentored and guided me throughout the AI Residency - it’s been a life-changing two years! My research mentors: Doug Eck, Jesse Engel, Adam Roberts and Fjord Hawthorne. Larger Magenta collaborators: Anna Huang, Monica Dinculescu, Ian Simon, Carey Radebaugh, Andy Coenen. DeepMind collaborators: Chenjie Gu. Tone Transfer team and AIUX: Nida Zada, Michelle Carney, Chong Li, Mark Bowers, Edwin Toh, Justin Secor. Sounds of India team: Miguel de Andres-Clavera, Yiling Liu, David Zats, Ping Yu, Aditya Mirchandani, KC Chung, Kiattiyot (Boon) Panichprecha. Google AI Residency Program Managers: Katie Meckley, Sally Jesmonth and Phing Lee. And so many more!